With the advent of large language models, we’ve all taken a fresh look at our data strategy. We find it hard to imagine the data strategy and data architecture even more than a tech strategy and setup. As Cassie Kozyrkov points out on datasets and decision intelligence, just collecting all data and trying to get something magical done by algorithms is going to take a lot of work, and it needs strategic decisions on data, setup, and rollout. Due to the focus and scale of this, we need to rethink how we approach our data strategy.
Dutch Grand Prix 2023
The Dutch GP in Zandvoort gave us an unexpected opportunity to see (part of) a data strategy interlinked in a physical setup. Most of the F1 setup is extremely visible, and due to sporting regulations and circuit setup (think Monaco, but also Zandvoort), there is a tight cap on resources and space. So, it is an excellent opportunity to learn from how these teams are making their decisions.
A tour of the Alpine pit box started at the most visible part — where the cars are prepared, and the drivers wait in their cars, watching the double screens placed on the hood, turning from 300 km/h drivers to analysts.
But less visible parts are even more interesting — around 20-30% of the precious space in the pit box is not accessible and has no windows. A sneak peak (no cameras allowed! 😉 ) revealed rows of terminals for data analysis. So, teams dedicated much of their cramped space for on-site analysis.
F1, in contrast to the teams, has chosen to centralize the heavy lifting of the data in a centralized setup in the UK, combining audio, visuals, and predictions before distributing it. It will be interesting to see how this setup keeps evolving and whether it will work in the coming years.
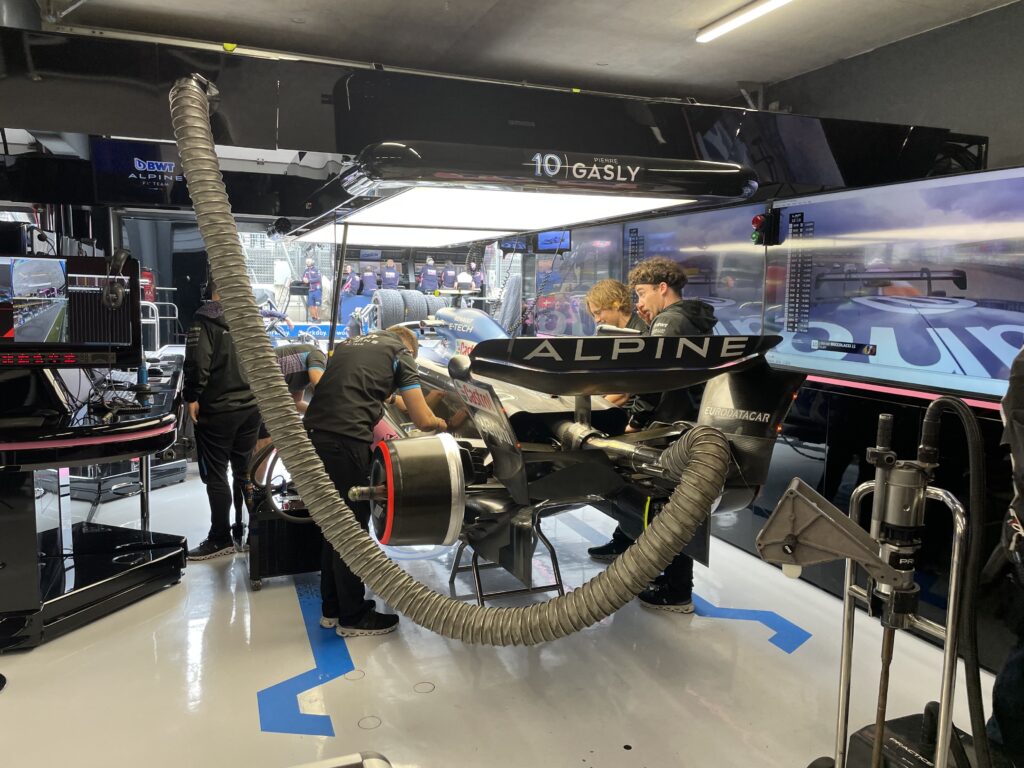
The pit wall
The Ferrari pit wall for the Melbourne GP of 2022 shows the evolution. Different disciplines have their own seat; screens and buttons for direct communication take up most real estate. The only one looking out is a cameraman.
Of course, this has been triggered by the data and IoT that F1 underwent. AWS states that 300 sensors on each F1 race car generate more than 1.1 million data points per second.
There is an exciting interview about the pit wall with Laurent Mekies, the race director for Ferrari in 2000.
He explains being on the wall gives an edge as they see, hear and feel the action and do not get lost in numbers. Interestingly, the race engineers are in the garage close to the car during other sessions than the race. The race engineer for Max is Gianpiero Lambiase, known from the interactions. During the race, they would feel lost in the garage, so they can be found on the pit wall. But Laurent also has this to say about the pit wall (1):
Of course, there is definitely a legacy aspect to it but it’s a legacy we’re all attached to because – and people sometimes forget this – we’re Formula 1 fans too. This is how it is supposed to be in F1.
Laurent Mekies, Ferrari Sporting Director
Another part of the pit setup might provide insights and an answer to the different evolved choices: the pit wall.
Ferrari’s pit wall for the 1985 Portuguese GP tells an exciting story — some Ferrari umbrellas, a Longines timing device, a board and an open view of the track. Everyone is focused on the track and the single monitor.
Fast forward fifteen years to a different track, and the change is evident. More screens are visible as Alain Prost and Nick Heidfeld check training times for the Spanish GP. Direct view and connection to the track are still part of the setup.
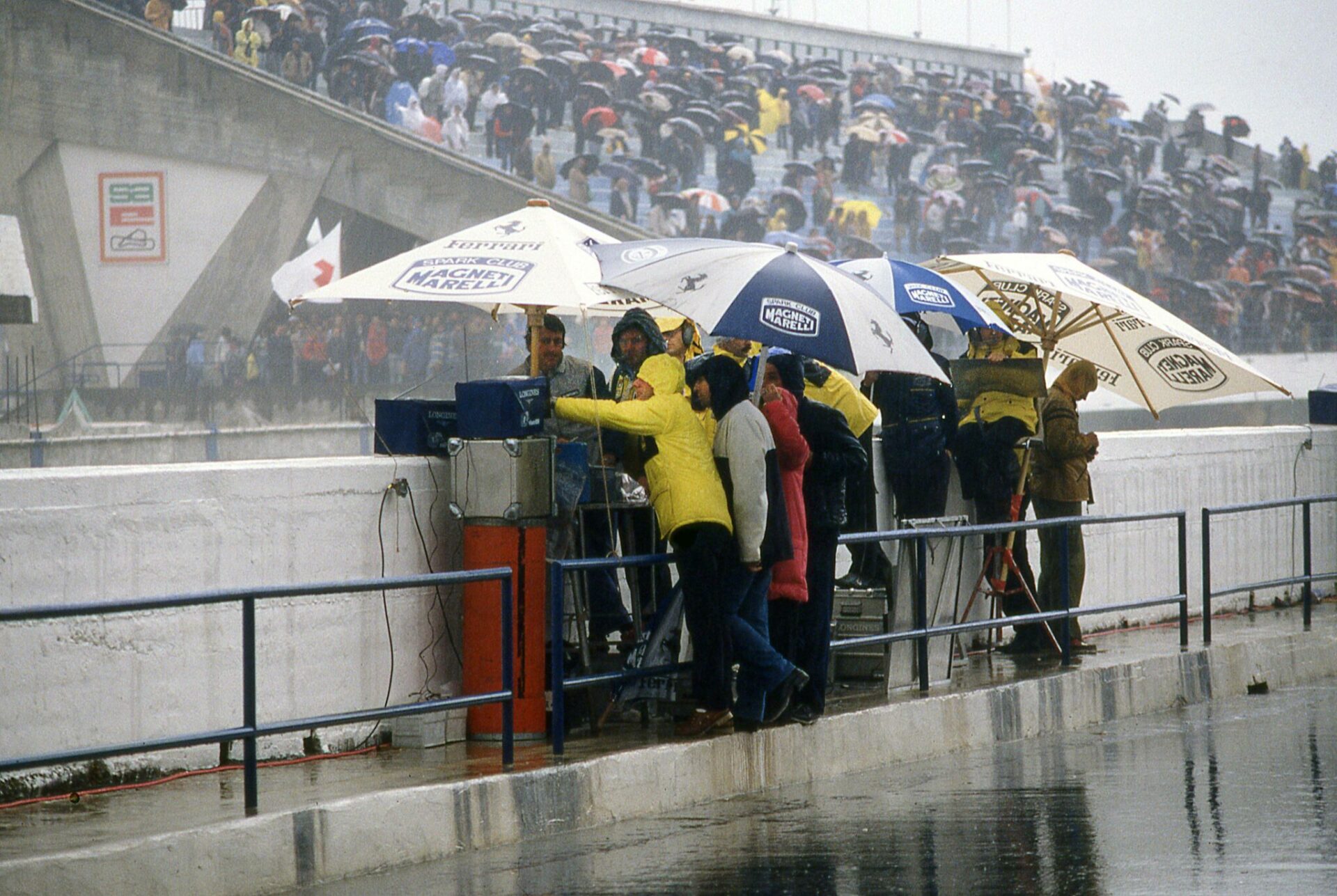
Given the budget cap, it will be interesting to follow what will happen: For 2023, Haas has decided to take a different approach; a smaller setup saves quite some budget. As you can see, Haas will spend that wisely (although not on data ;-)).
Will this evolve into its natural conclusion? A wall where the focus is on track and data is interpreted elsewhere. We might also see other data aggregation tools (Apple vision? AR? Or a small handheld?
Relating this to our own decision intelligence, Insights, and reports start as an idea like the wall in 1985: small, MVP but not optimal. Over time, the report, script or database grows in visibility and importance. Scope and importance change, but the general shape or form is not challenged.
With the growing budgets for data and the importance, we forget to challenge and see what is there.
We need to create a budget cap to evoke the type of move that Haas did to see if we can take the first steps to reshape and rethink. As with the pit wall, reports and insights carry a lot of costs in preparing and maintaining that are not visible most of the time.
Big data
Returning to the big, closed room points to another development. The data collection has changed, and the data solutions around F1 and the world have evolved. Algorithms and predictions have replaced predictable, stable and standardized models generated by nightly jobs and queried by scheduled reports.
While data analytics and data science were always ready, with the cloud boosting the data engineering workloads, the door is open for innovation using data. All major tools for data analysis focus nowadays on this mindset providing countless connectors for easy integration and usually a web page where data can be intuitively squeezed of all its meaning, more and more along with an AI assistant.
If the previous generation of data solutions could only be used post- race by the teams looking for insights on how the pilot and the machine performed, current technology allows not only to collect and analyze data in real time with the goal of fine tuning parameters to extent the car’s maximum performance window but also to create predictions on how the car will perform throughout the entire season on different circuits with the goal of maximizing the window of opportunity for each specific track layout.
That has triggered the teams to dedicate more and more prime real estate to the interpreters and builders, so as to have these as part of the teams. But will that be fast and innovative enough to take full use? McLaren goes about this differently. The company has a small data science team and tries to create citizen data scientists and give everyone in their setup (800 in total) access to the data and the algorithms and insights (2).
So we see teams experimenting with opening that guarded black box to a larger part of the organization to leverage these real time models. Will that mean that the TV screens in the pit box will also become interactive for the mechanics? And how do you keep that from the rest of the world? (3)
Answering your questions
A data strategy cannot be successful in its purpose to answer the question of what the organization will do with the data to be more successful in the absence of a solid Data Governance component.
Real Time Diagnostic Analytics and Near Real Time Predictive and Prescriptive Analytics are enablers in the race to be the fastest and most innovative, enabling data citizens is a huge step towards being a data driven organization; however, without the backbone of governance, no data organism will be resilient enough to evolve and withstand time.
This is exactly the discussion we need to have as well. How do we propagate these real time models? How do we share data in the organization and not outside? Do we want a closed off room or citizen scientists?
Let’s see if we see these data walls appear in the garages or that parts of the garage will be shielded in order to create this integration. But in the coming seasons, we’re going to watch these setups as we do with the pit boxes.